iCoSys has opened a new axis of applied research projects in the field of ICT for Industry 4.0. Born in April 2017, it aims at providing applied computer science solutions to foster the development of Industry 4.0 systems.
The Smart Factory Group in iCoSys is thus active in many domains, including:
- industrial big data and analytics for anomaly detection, smart manufacturing and predictive maintenance
- industrial machine learning
- industrial mobile applications
- information indexing linked to production sites
- simulation, digital twins
Projects
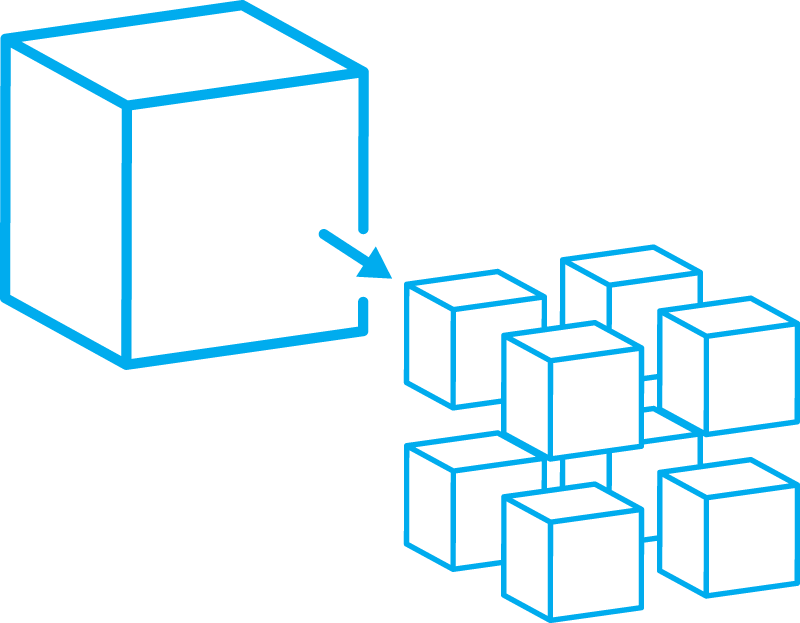
At iCoSys Fribourg, we ave set up a microservice cluster to deploy machine learning models scheduled by Kubernetes, including shared GPU support for deep learning.
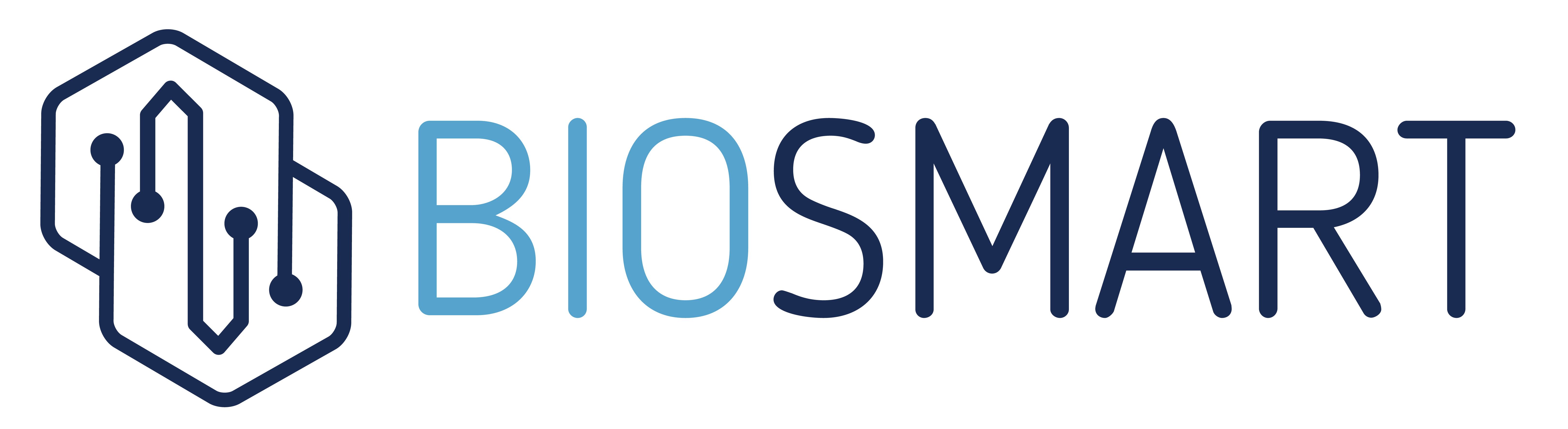
The European project BIOSMART aims to develop active and smart bio-based and compostable packages that make possible: light weighting, reduced food residues, shelf life monitoring, longer shelf life and easier consumer waste handling.
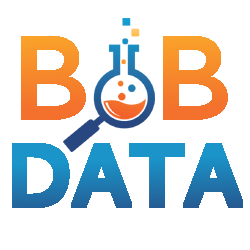
BBDATA stands for Big Building Data and aims at developing a scalable cloud platform and tools for storing and processing smart building data. The services are targeting data access, processing and analysis, using open, robust, standardized and secured big data technologies.
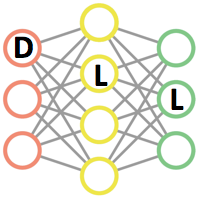
DLL is a machine learning framework that aims to provide a C++ implementation of neural networks and convolutional neural networks. The implementation provides significant speedups on cpu in comparison to other frameworks such as TensorFlow or Torch. It is then dedicated to the use phase of such deep models where performance on cpu is requested, such as in industry 4.0 applications.
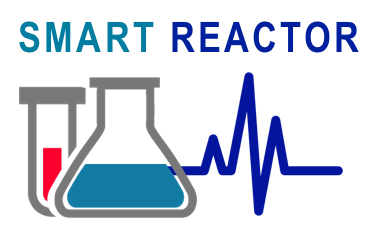
In this project, iCoSys is specifically interested in the analysis and development of versatile approaches for the detection of abnormal events using machine learning approaches (WP5).
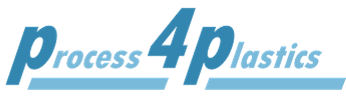
Process4Plastics (P4P) aims to build a dedicated methodology of process engineering for the production of plastics parts. In the industry 4.0 era, machines will be equipped with many sensors and actuators and be connected together to create intelligent networks.
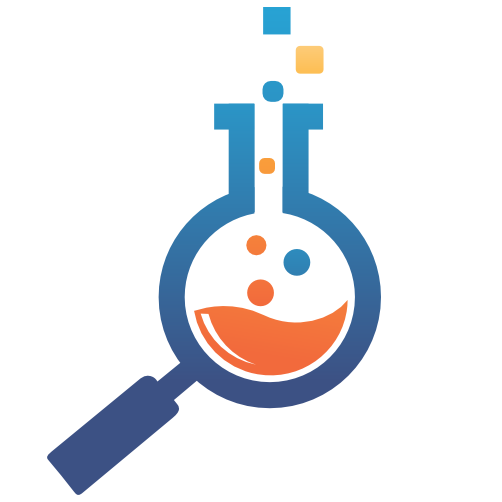
DAPLAB aims at facilitating the access for enterprises and universities to emerging technologies in the area of big data and intelligent data analysis by managing an infrastructure composed of cluster of servers dedicated to storage and computation, to which universities and local companies will have access.
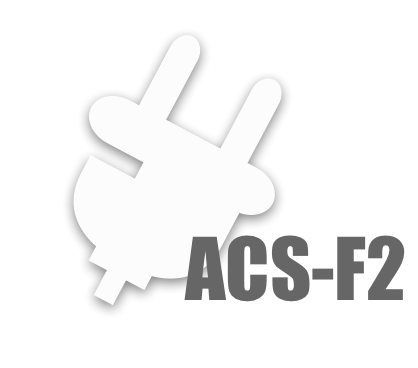
This machine learning dataset contains electric consumption signatures of 225 appliances, spread into 15 categories and including 6 time series such as current, active power and reactive power. It also includes two test protocols to be used for appliance recognition tasks.
All projects here.