OptiSoil Artificial intelligence applied to the process of excavation for buildings construction
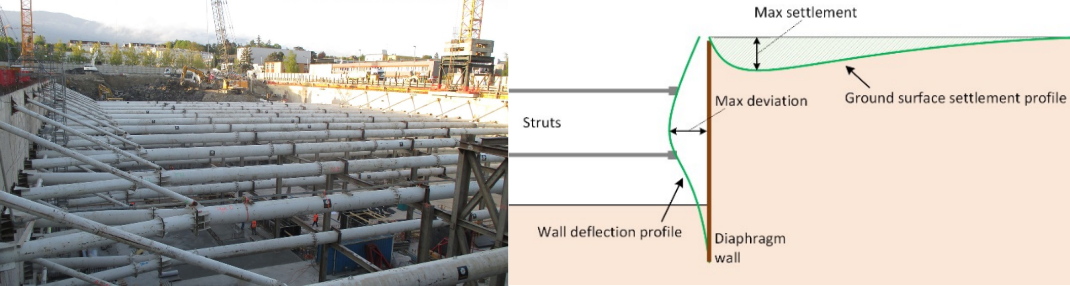
In several technical domains, such as mechanics or civil engineering, numerical simulation programs based on finite elements allows to simulate the behavior of the studied components. Very often, a full numerical simulation is necessary when, eventually, only a few values are beneficial to engineers. It is the case in the process of excavations for building construction. In this field, the ZSWalls simulation software is known to be able to predict some parameters such as internal forces in the support system (concrete or steel wall, anchors) and associated displacements accurately, given the geometry of the excavation, the stratigraphy, the water level, and the support system itself.
We propose here to take advantage of this software to build a synthetic database composed of thousands of excavations and then use machine learning to predict the useful parameters of a new case. The main advantage of this approach is that the trained model is much faster to infer prediction than running a complete numerical simulation.
Our main innovations are:
- Reproduction of significant numerical simulation results using deep learning and more precisely Convolutional Neural Network – CNN.
- Data-sets generation through numerical simulation – as opposed to the traditional method of data collection. Any field using a numerical simulation could actually benefit from this method.
- Transformation of numerical data describing an excavation to a CNN-compatible format (image-like data).
We aim at developing prototype web applications that allows civil engineers to automatically calculate the optimal way to approach a specific excavation, leading to significant time and cost savings.
Publications
-
S. Commend, S. Wattel, J. Hennebert, P. Kuonen, and L. Vulliet, “Prediction of unsupported excavations behaviour with machine learning techniques,” in COMPLAS 2019, 2019, pp. 529-535.
[Bibtex]@InProceedings{commend2019prediction, author={St{\'{e}}phane Commend and Sacha Wattel and Jean Hennebert and Pierre Kuonen and Laurent Vulliet}, booktitle={COMPLAS 2019}, title={Prediction of unsupported excavations behaviour with machine learning techniques}, year={2019}, pages={529-535}, month={September}, }
-
B. Wolf, J. Donzallaz, C. Jost, A. Hayoz, S. Commend, J. Hennebert, and P. Kuonen, “Using CNNs to Optimize Numerical Simulations in Geotechnical Engineering,” in Artificial Neural Networks in Pattern Recognition, Cham, 2020, p. 247–256.
[Bibtex]@InProceedings{10.1007/978-3-030-58309-5_20, author="Wolf, Beat and Donzallaz, Jonathan and Jost, Colette and Hayoz, Amanda and Commend, St{\'e}phane and Hennebert, Jean and Kuonen, Pierre", editor="Schilling, Frank-Peter and Stadelmann, Thilo", title="Using CNNs to Optimize Numerical Simulations in Geotechnical Engineering", booktitle="Artificial Neural Networks in Pattern Recognition", year="2020", publisher="Springer International Publishing", address="Cham", pages="247--256", abstract="Deep excavations are today mainly designed by manually optimising the wall's geometry, stiffness and strut or anchor layout. In order to better automate this process for sustained excavations, we are exploring the possibility of approximating key values using a machine learning (ML) model instead of calculating them with time-consuming numerical simulations. After demonstrating in our previous work that this approach works for simple use cases, we show in this paper that this method can be enhanced to adapt to complex real-world supported excavations. We have improved our ML model compared to our previous work by using a convolutional neural network (CNN) model, coding the excavation configuration as a set of layers of fixed height containing the soil parameters as well as the geometry of the walls and struts. The system is trained and evaluated on a set of synthetically generated situations using numerical simulation software. To validate this approach, we also compare our results to a set of 15 real-world situations in a t-SNE. Using our improved CNN model we could show that applying machine learning to predict the output of numerical simulation in the domain of geotechnical engineering not only works in simple cases but also in more complex, real-world situations.", isbn="978-3-030-58309-5", url={https://link.springer.com/chapter/10.1007/978-3-030-58309-5_20}, doi={https://doi.org/10.1007/978-3-030-58309-5_20} }