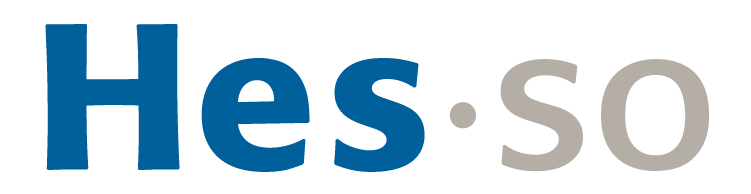
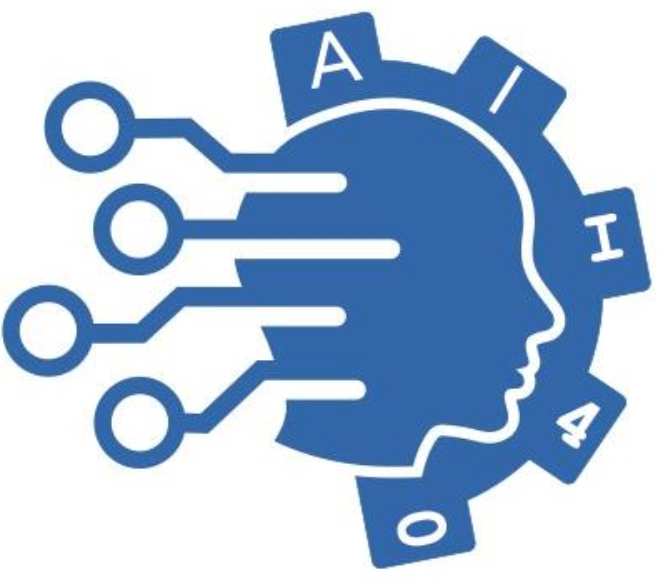
Problem
Swiss manufacturing industries will have to rely on innovation to stay competitive at the international level. As a high-wage exporting country, Switzerland must offer strong added value products in order to consolidate its international market positions. With the rise of Industry 4.0 paradigms, opportunities are now opening for industrial process monitoring, continuous quality inspection and predictive maintenance. In this context, the analysis of data emitted by sensors using Machine Learning (ML) methods has a significant potential to optimize production efficiency by reducing costs while maintaining quality.
However, we see several challenges when setting up such ML systems, which represents a major barrier to an adoption in industrial applications:
- Data collection : The diversity and fragmentation of digital “ecosystems” sitting close to production machines makes the connection to machines and data collection a tedious and laborious manual task.
- Edge AI : For security and/or real-time constraints, edge platforms are becoming important to run AI applications. Current ML techniques, mostly conceived for cloud platforms, must be adapted.
- ML technical complexity : In order to unlock the full potential of AI in industry, advanced ML techniques like supervised approaches must be considered. These techniques still need a large amount of expertise and manual tuning to determine adequate settings.
- ML robustness to changing conditions : Industrial contexts are often fast evolving with, for example, upgrades of production chain or seasonal effects, tampering the quality of ML models that need to be frequently updated. Horizontal scalability of the data pipelines is also needed for large deployment.
Solution
The project AII4.0 aims at solving the previously mentioned challenges towards more standardized, universal and scalable AI for predictive maintenance solutions. More specifically, the innovation is focused on:
- Building standards for “on-premises” data collection through IoT and edge AI execution.
- Going beyond state-of-the-art ML approaches currently used for industrial process monitoring with data augmentation, self-supervised/active-learning, self-tuning AutoML.
- Extending DataOps and MLOps techniques for industrial context in order to ensure safe ML model deployments, scalable ML model re-training and human-in-the-loop inclusion.
Value proposition
Production machines have structures and properties that are dedicated to various fields of application like in watch industry, pharmaceutical industry and food technologies. Pushed by democratization and standardization of sensor technologies (IoT), the monitoring of these systems is becoming more and more intense. We want to propose AI solutions relying on Machine Learning for superior machine monitoring and predictive maintenance. Such approaches bring economic benefits in comparison to simulation-based solutions traditionally used to build digital twins. First, ML models are data-driven, making them adaptable to various industrial contexts and therefore less expensive to build. Second, ML models include many trainable parameters and rely on less hypotheses than statistical approaches, making them more precise and robust to noise. Finally, the recent democratization of hardware acceleration (GPUs), edge computation and DevOps strategies allows for scalability, data protection and economic viability of such offerings.