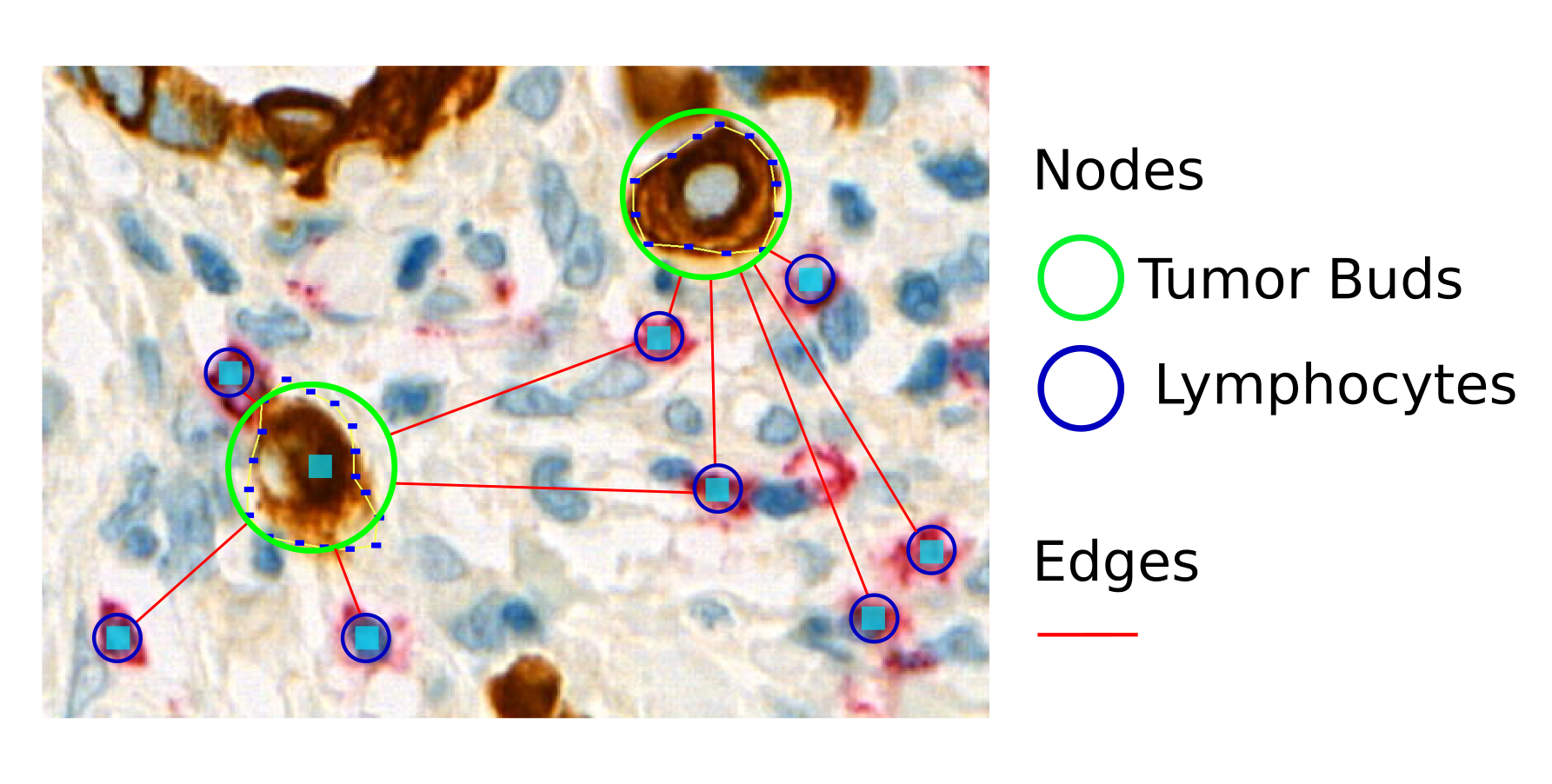
Colorectal cancer (CRC) is the third most common malignancy in Switzerland and remains lethal in almost half of newly diagnosed patients. The most important parameter for prognosis and determining patient management is tumor spread according to the Tumor-Nodal- Metastasis (TNM) classification. However, the TNM system may show considerable differences in tumors within the same stage. This is particularly evident for stage II tumors, where 5-year survival ranges from 37%-67% 1. Therefore, additional biomarkers are needed for improved risk stratification in certain CRC patient subsets.
Tumor budding, the presence of single tumor cells and small clusters of tumor cells at the invasive tumor front, has been proposed to be such a biomarker. However, tumor buds are only part of the tumor micro-environment and do not account for other factors, such as the biological host response. Especially T-cell infiltrates have been extensively examined as a protective biomarker, leading to ‘Immunoscore’, a combined digital assessment of the density of CD3+/CD8+ T-cells in the center and the periphery of CRC. Our own preliminary data supports the above-mentioned study that a combined assessment of tumor budding and T-cell infiltrates as well-established biomarkers in CRC may be superior to either marker alone. So far the BTS (Budding-T-cell-Score) was calculated from each area as the average buds among all punches divided by the average of T-cell counts among all punches.
In this project, we want to further investigate the BTS score using graph-based deep learning techniques. In a first step, we need to detect tumor buds and lymphocytes on PanCK-stained whole slide images (WSIs). Secondly, graph-based representations of the WSIs are created based on the tumor buds and T-cells. Graphs allow modeling parts of an object with nodes and relationships between the nodes with edges. In our case, graphs will be used to model the structure of cells or cell regions. We believe that the graph-based representation will be beneficial for the combined assessment of tumor buds and T-cells, because it captures not only the raw count of the cells but also their structural arrangement, which, we hypothesize, might play a central role for predicting clinical endpoints. In a third step, geometric deep learning (see also http://geometricdeeplearning.com), which extends deep learning beyond the domain of Euclidean geometry, is applied to the graphs to predict clinical endpoints, such as survival time.
Project Publications
- Linda Studer, Annika Blank, John-Melle Bokhorst, Iris Nagtegaal, Inti Zlobec, Alessandro Lugli, Andreas Fischer, Heather Dawson, “Taking tumour budding to the next frontier—a post International Tumour Budding Consensus Conference (ITBCC) 2016 review”, published in the Journal of Histopathology [Website]
[PDF]
[Bibtex]
@article{studer2021taking, title={Taking tumour budding to the next frontier—a post International Tumour Budding Consensus Conference (ITBCC) 2016 review}, author={Studer, Linda and Blank, Annika and Bokhorst, John-Melle and Nagtegaal, Iris D and Zlobec, Inti and Lugli, Alessandro and Fischer, Andreas and Dawson, Heather}, journal={Histopathology}, volume={78}, number={4}, pages={476--484}, year={2021}, publisher={Wiley Online Library} }
- Linda Studer, John-Melle Bokhorst, Inti Zlobec, Alessandro Lugli, Andreas Fischer, Francesco Ciompoi, Jeroen van der Laak, Iris Nagtegaal and Heather Dawson, “Validation of computer-assisted tumour-bud and T-cell detection in pT1 colorectal cancer”, published at the 32 nd Congress of the ESP and XXXIII International Congress of the IAP (Oral Free Paper Session at the One-Day Computational Pathology Symposium) [Website]
[PDF]
[Bibtex]
@article{studer2020validation, title={Validation of computer-assisted tumour-bud and T-cell detection in pT1 colorectal cancer}, booktitle={32 nd Congress of the ESP and XXXIII International Congress of the IAP}, author={Studer, Linda and Bokhorst, John-Melle and Zlobec, Inti and Lugli, Alessandro and Fischer, Andreas and Ciompi, Francesco and van der Laak, Jeroen and Nagtegaal, Iris and Dawson, Heather}, year = {2020}, volume = {477}, number = {1}, pages = {S37-S38}, DOI = {https://doi.org/10.1007/s00428-020-02938-x}, journal = {Virchows Archiv, European Journal of Pathology}, publisher = {Springer} }
- Linda Studer, John-Melle Bokhorst, Inti Zlobec, Alessandro Lugli, Andreas Fischer, Francesco Ciompoi, Jeroen van der Laak, Iris Nagtegaal and Heather Dawson, “Quality comparison of computer-assisted T-cell and tumor-bud detection in pT1 colorectal cancer from four different pathology institutions” published in Der Pathologe as part of the 86th annual congress of the Swiss Society of Pathology [Website][PDF]
[Bibtex]
@article{studer2020quality, author = "Studer, Linda and Bokhorst, John-Melle and Zlobec, Inti and Lugli, Alessandro and Fischer, Andreas and Ciompi, Francesco and van der Laak, Jeroen and Nagtegaal, Iris and Dawson, Heather", title = "Quality comparison of computer-assisted T-cell and tumor-bud detection in pT1 colorectal cancer from four different pathology institutions", journal = "Der Pathologe", volume = "41", number = "6", pages = "682", year = "2020", DOI = "https://doi.org/10.1007/s00292-020-00849-x", publisher="Springer Medizin" }
- Linda Studer, John-Melle Bokhorst, Francesco Ciompi, Andreas Fischer, Heather Dawson, “The BT-Hotspot Graph Dataset: Investigating the Relation of Tumor-Buds and T-Cells in Colorectal Cancer Tumor Budding Hotspots”, published at the “What Did We Expected from Porto’s ECDP2020?” in Journal of Pathology Informatic
[PDF]
[Bibtex]
@article{studer2020bthotspot, title={The BT-Hotspot Graph Dataset: Investigating the Relation of Tumor-Buds and T-Cells in Colorectal Cancer Tumor Budding Hotspots}, author={Linda Studer, John-Melle Bokhorst, Francesco Ciompi, Andreas Fischer, Heather Dawson}, journal={"What Did We Expected from Porto's ECDP2020?" in Journal of Pathology Informatics}, volume={11}, year={2020}, publisher={Wolters Kluwer--Medknow Publications} }
Related Publications
- Christian Abbet, Linda Studer, Andreas Fischer, Heather Dawson, Inti Zlobec, Behzad Bozorgtabar, Jean-Philippe Thiran, “Self-Rule to Adapt: Learning Generalized Features from Sparsely-Labeled Data Using Unsupervised Domain Adaptation for Colorectal Cancer Tissue Phenotyping”, published at Medical Imaging with Deep Learning 2021 [Website]
[PDF]
[Bibtex]
@inproceedings{ abbet2021selfrule, title={Self-Rule to Adapt: Learning Generalized Features from Sparsely-Labeled Data Using Unsupervised Domain Adaptation for Colorectal Cancer Tissue Phenotyping}, author={Christian Abbet and Linda Studer and Andreas Fischer and Heather Dawson and Inti Zlobec and Behzad Bozorgtabar and Jean-Philippe Thiran}, booktitle={Medical Imaging with Deep Learning}, year={2021}, url={https://openreview.net/forum?id=VO7asaS5GUk} }
- Linda Studer, Jannis Wallau, Heather Dawson, Andreas Fischer, “Graph-based Classification of Intestinal Glands in Colorectal Cancer Tissue Images”, published at the 2020 25th International Conference on Pattern Recognition (ICPR)
[PDF]
[Bibtex]
@inproceedings{studer2021classification, title={Classification of Intestinal Gland Cell-Graphs Using Graph Neural Networks}, author={Studer, Linda and Wallau, Jannis and Dawson, Heather and Zlobec, Inti and Fischer, Andreas}, booktitle={2020 25th International Conference on Pattern Recognition (ICPR)}, pages={3636--3643}, year={2021}, organization={IEEE} }
- Linda Studer, Jannis Wallau, Rolf Ingold, Andreas Fischer, “Effects of Graph Pooling Layers on Classification with Graph Neural Networks”, published at the 2020 7th Swiss Conference on Data Science (SDS)
[PDF]
[Bibtex]
@inproceedings{studer2020effects, title={Effects of Graph Pooling Layers on Classification with Graph Neural Networks}, author={Studer, Linda and Wallau, Jannis and Ingold, Rolf and Fischer, Andreas}, booktitle={2020 7th Swiss Conference on Data Science (SDS)}, pages={57--58}, year={2020}, organization={IEEE} }
- Linda Studer, Jannis Wallau, Heather Dawson, Inti Zlobec, Andreas Fischer, “Intestinal Gland Classification Using Graph Neural Networks”, published at the “What Did We Expected from Porto’s ECDP2020?” in Journal of Pathology Informatic
[PDF]
[Bibtex]
@article{studer2020intestinal, title={Intestinal Gland Classification Using Graph Neural Networks}, author={Linda Studer, Jannis Wallau, Heather Dawson, Inti Zlobec, Andreas Fischer}, journal={"What Did We Expected from Porto's ECDP2020?" in Journal of Pathology Informatics}, volume={11}, year={2020}, publisher={Wolters Kluwer--Medknow Publications} }
- Linda Studer, Sven Wallau, Heather Dawson, Inti Zlobec, Andreas Fischer, “Reducing the Annotation Workload with Transfer Learning: a Feasibility Study for Intestinal Gland Segmentation”, published at the “What Did We Expected from Porto’s ECDP2020?” in Journal of Pathology Informatic
[PDF]
[Bibtex]
@article{studer2020reducing, title={Reducing the Annotation Workload with Transfer Learning: a Feasibility Study for Intestinal Gland Segmentation}, author={Linda Studer, Sven Wallau, Heather Dawson, Inti Zlobec, Andreas Fischer}, journal={"What Did We Expected from Porto's ECDP2020?" in Journal of Pathology Informatics}, volume={11}, year={2020}, publisher={Wolters Kluwer--Medknow Publications} }
- Linda Studer, Shushan Toneyan, Inti Zlobec, Heather Dawson, Andreas Fischer, “Intestinal Gland Classification from Colorectal Cancer Tissue Images using Graph-based Methods” published in Der Pathologe as part of the 4th Joint Annual Meeting of the Swiss and Austrian Societies of Pathology [Website]
[Bibtex]
@article{studer2019intestinal, author = "Studer, Linda and Toneyan, Shushan and Zlobec, Inti and Lugli, Alessandro and Fischer, Andreas and Dawson, Heather", title = "Intestinal Gland Classification from Colorectal Cancer Tissue Images using Graph-based Methods", journal = "Der Pathologe", volume = "40", number = "6", pages = "688-689", year = "2019", DOI = "https://doi.org/10.1007/s00292-019-00679-6", issn = "1432-1963", publisher="Springer Medizin" }
- Linda Studer, Shushan Toneyan, Inti Zlobec, Heather Dawson, Andreas Fischer, “Graph-based Classification of Intestinal Glands in Colorectal Cancer Tissue Images”, published at the Computational Pathology Workshop COMPAY, MICCAI 2019 [Website]
[PDF]
[Bibtex]
@inproceedings{studer2019graph, title={Graph-based Classification of Intestinal Glands in Colorectal Cancer Tissue Images}, author={Studer, Linda and Toneyan, Shushan and Zlobec, Inti and Dawson, Heather and Fischer, Andreas}, year={2019}, booktitle = {2nd COMPAY Workshop, 22nd International Conference on Medical Image Computing and Computer-Assisted Intervention (MICCAI)}, year={2019}, }
Datasets
- pT1 Gland Graph Dataset on GitHub